Best Practice Sampling Bias in Research: How to Avoid it
Introduction
Sampling bias poses a significant threat to the validity of research findings by distorting results toward a particular segment of a target population. This article introduces the concept of sampling bias, highlighting its presence in various fields such as healthcare, education, psychology, and marketing. It explores the causes and impacts of sampling bias, outlines its different types, and provides researchers with the necessary tools to detect and mitigate this pervasive issue.
By offering strategies to reduce and avoid sampling bias, the goal is to improve the credibility and trustworthiness of research outcomes, ensuring they more accurately represent the entire population under study.
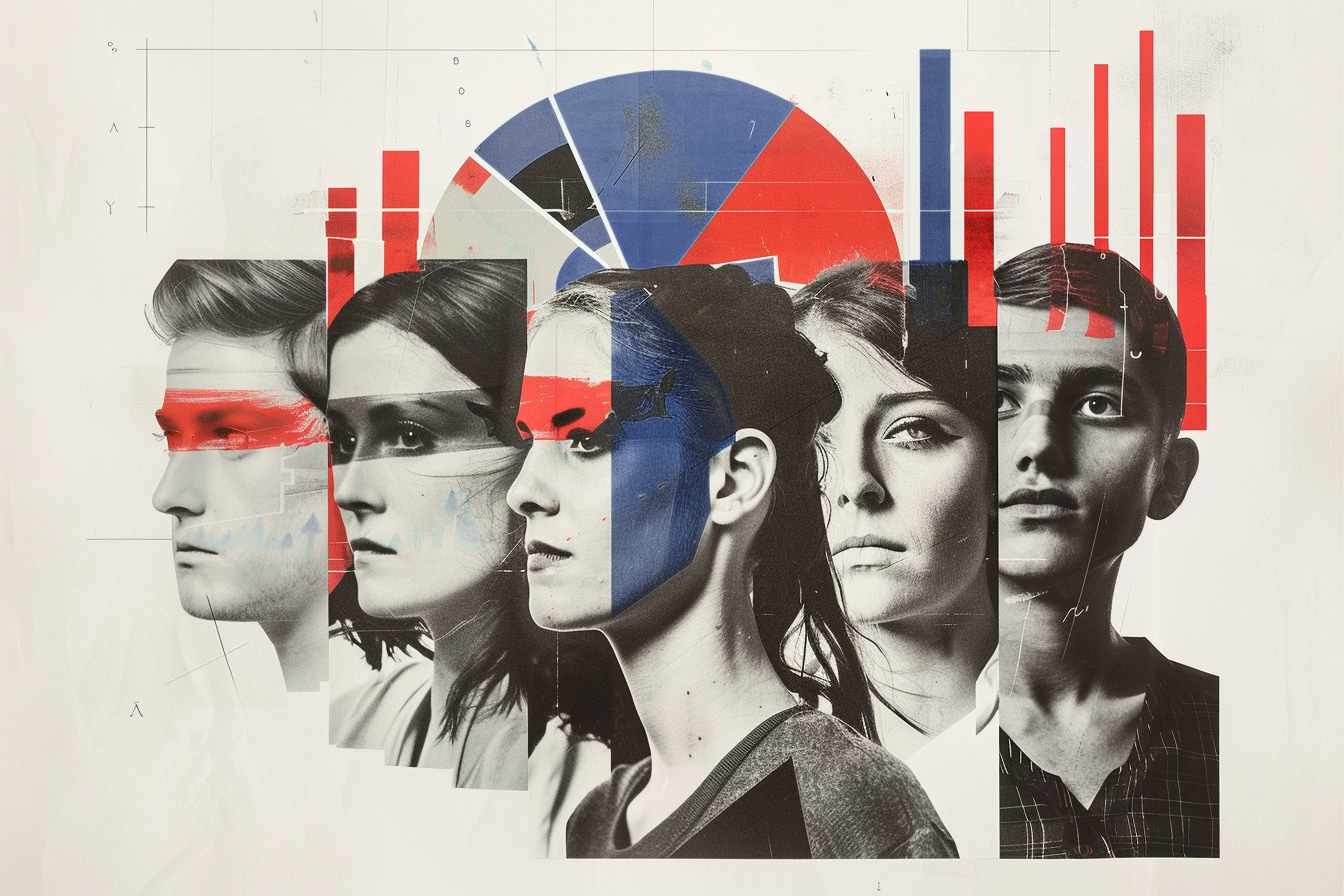
What is sampling bias?
Sampling bias occurs when the process used to select participants or data points for a study leads to a sample that is not representative of the population from which it was drawn. This non-representativeness can skew the research findings, making them less generalizable and potentially misleading.
The essence of sampling bias lies in the systematic exclusion or over-representation of certain groups within the population. For example, if a study on workplace productivity only includes participants from urban areas, ignoring rural workers, the conclusions may not accurately reflect the broader workforce. Similarly, online surveys might inadvertently favor younger, more tech-savvy respondents, leaving out older demographics or those with limited internet access.
A biased sample can manifest in various forms, depending on the method of sample selection. Convenience sampling, where participants are chosen based on their accessibility to the researcher, often leads to sampling bias because it does not consider the diversity of the population. Voluntary response bias, another form, occurs when individuals choose themselves to participate, which can result in a sample with stronger opinions or interests than the general population.
The consequences of biased samples extend beyond the accuracy of research findings; they can also impact policy decisions, resource allocation, and scientific understanding. For instance, medical research that fails to include diverse ethnic groups may overlook important variations in health outcomes or treatment efficacy. Therefore, identifying and addressing sampling bias contributes to the integrity and applicability of research outcomes, ensuring they are valid and valuable for the broader population.
What are some examples of sampling bias?
Sampling bias can affect various fields of study, leading to skewed results and potentially flawed conclusions. Below are examples from healthcare, education, psychology, and marketing, illustrating how sampling bias can manifest in different contexts.
Healthcare
In a study aimed at evaluating the effectiveness of a new heart disease medication, researchers decide to recruit participants from a single, high-income urban hospital. This decision inadvertently excludes a significant portion of the population, particularly those from lower-income backgrounds and rural areas, who may have different health profiles and access to healthcare.
As a result, the findings may not accurately represent the medication’s effectiveness across the broader population, potentially overlooking variations in drug efficacy or side effects experienced by different demographic groups.
Education
Consider a research project investigating the impact of digital learning tools on student performance. If the study primarily involves schools with advanced technological resources, it may not account for schools in areas where such tools are scarce.
This exclusion leads to a sampling bias that paints an incomplete picture of the digital learning tools’ effectiveness, failing to consider the challenges and benefits experienced by students in a more diverse range of educational environments.
Psychology
A psychologist conducting a study on stress management techniques uses social media to recruit participants. This approach is likely to attract individuals who are not only active on these platforms but also those who have a particular interest in stress management.
Consequently, the sample may not adequately represent the general population’s stress levels or coping mechanisms, skewing the results towards those already predisposed to seeking out stress management strategies.
Marketing
A company launching a new product decides to gather consumer feedback by distributing surveys at an upscale shopping mall. This method primarily captures the opinions of shoppers with higher spending power, neglecting potential customers from various other socioeconomic backgrounds.
The feedback collected is biased towards the preferences and attitudes of a wealthier demographic, which may not reflect the broader consumer base’s views and could mislead the company in its marketing strategies.
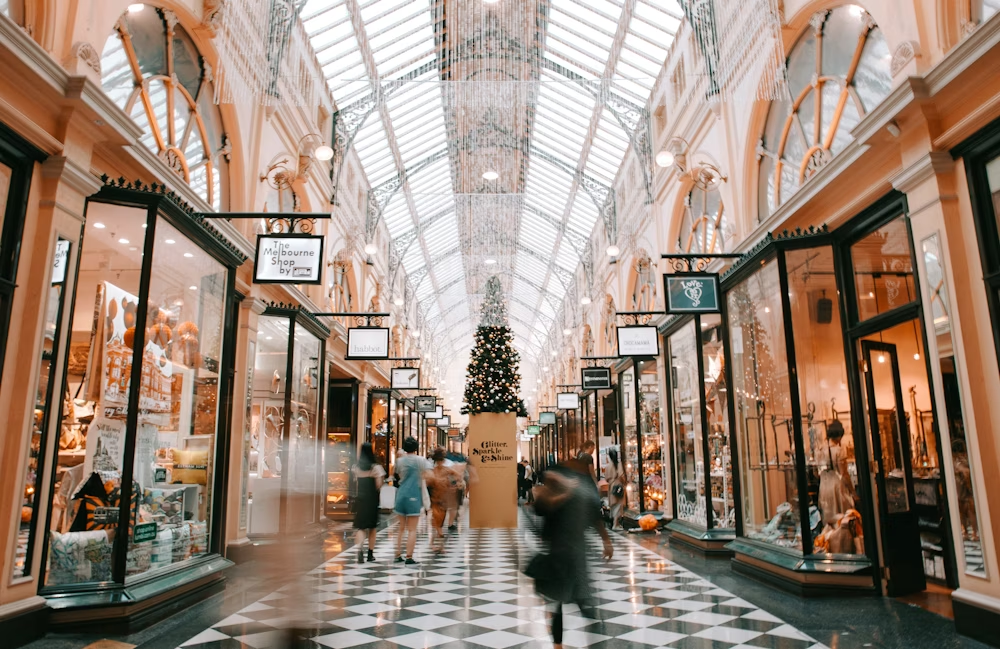
What causes sampling bias?
Sampling bias arises from various factors, each contributing to the skewing of results in research. Understanding these causes is beneficial for researchers aiming to mitigate their impact. This section breaks down the major causes of sampling bias into distinct categories.
Selection process
One of the primary causes of sampling bias is the selection process used to choose participants or data points for a study. When this process is not random or does not account for the diversity of the population, certain groups may be systematically excluded or over-represented.
For instance, relying solely on volunteers can lead to a sample that is more motivated or interested in the research topic than the general population, known as voluntary response bias.
Accessibility
Accessibility issues also play a significant role in sampling bias. Studies that only include participants who are easy to reach, such as people living in urban areas or those who frequent certain institutions, can miss out on a wide range of perspectives.
For example, a survey conducted online might exclude individuals without internet access or those who are not tech-savvy, leading to an unrepresentative sample of the population.
Non-response
Non-response bias occurs when a significant portion of selected participants chooses not to respond or participate in the study.
The reasons for non-response can vary, including lack of interest, time constraints, or privacy concerns. The individuals who do not participate may differ in ways from those who do, potentially skewing the study’s results.
Researcher bias
Researcher bias refers to the conscious or unconscious preferences and assumptions held by researchers, which can influence the selection of study participants.
For example, a researcher might subconsciously choose participants who appear more cooperative or interested in the study topic. This can lead to a sample that does not accurately reflect the diversity of the population.
Sampling frame issues
The sampling frame—the list or database from which participants are chosen—can also contribute to sampling bias if it does not represent the target population. For instance, using a voter registration list to study public opinion might exclude non-registered voters, who could have different views from those on the list.