How to reduce sampling bias
How to reduce sampling bias
Reducing sampling bias is essential for enhancing the accuracy and reliability of research findings. By employing specific methodological approaches, researchers can obtain a more representative sample, ensuring that their study reflects the population of interest. This section outlines strategies to significantly reduce sampling bias, focusing on the sample selection process, data collection techniques, and specific considerations for minimizing recall bias.
Use random sampling
Random sampling is foundational for obtaining a representative sample. By giving every member of the population an equal chance of being selected, random sampling minimizes the risk of bias in the sample selection process.
This method counters the tendency to over-represent or under-represent specific characteristics of the population. Implementing random sampling can be as straightforward as using a random number generator to select participants from a list, and this random sample will then be statistically likely to reflect the broader population’s diversity.
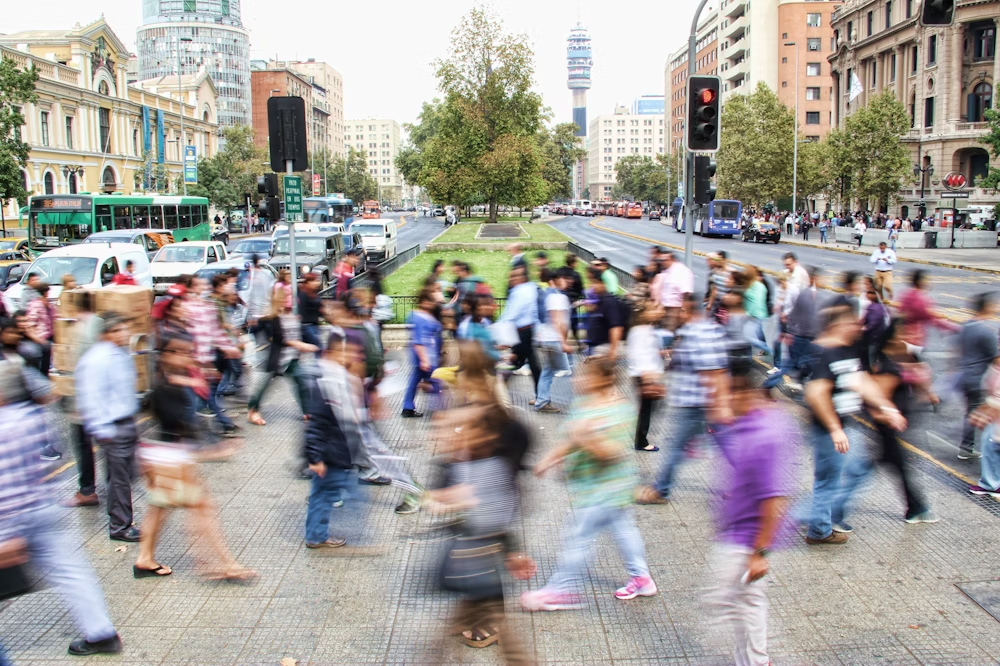
Implement stratified sampling
Stratified sampling enhances the representativeness of a sample by dividing the population into strata, or subgroups, based on key characteristics and then randomly selecting participants from each stratum.
This approach ensures that all segments of the population are included in the sample in proportion to their prevalence in the population. Stratified sampling is particularly effective in studies where specific characteristics are known to affect the research outcome, as it can significantly reduce sampling bias by guaranteeing that these characteristics are adequately represented.
Opt for systematic sampling
Systematic sampling involves selecting participants at regular intervals from an ordered list, combining the simplicity of random sampling with the added assurance of spread across the population.
After randomly choosing a starting point, researchers select every nth participant, with the interval n determined by the desired sample size and the size of the population. This method simplifies the sample selection process and can help distribute the selection evenly across the population.
Enhance data collection methods
To collect data more effectively and inclusively, researchers can employ multiple data collection methods, reaching out to participants through various channels. This multi-pronged approach ensures that different segments of the population, especially those that might be hard to reach through a single method, have the opportunity to participate in the study.
By diversifying how they collect data, researchers can mitigate biases associated with accessibility and non-response. Moreover, findings can be triangulated across the different data sources to verify that the findings are not biased by a single data source.
Address recall bias
To mitigate recall bias, researchers should design questionnaires and interviews that help all participants accurately recall information. This can involve using neutral language, providing cues or timelines to aid memory, and validating responses against other sources of data when possible.
Employing such techniques ensures that the accuracy of recollections does not disproportionately affect one group over another, reducing the impact of recall bias on the study’s findings.