Regression Analysis: A Complete Guide to Understand
Regression Analysis: A Complete Guide to Understand
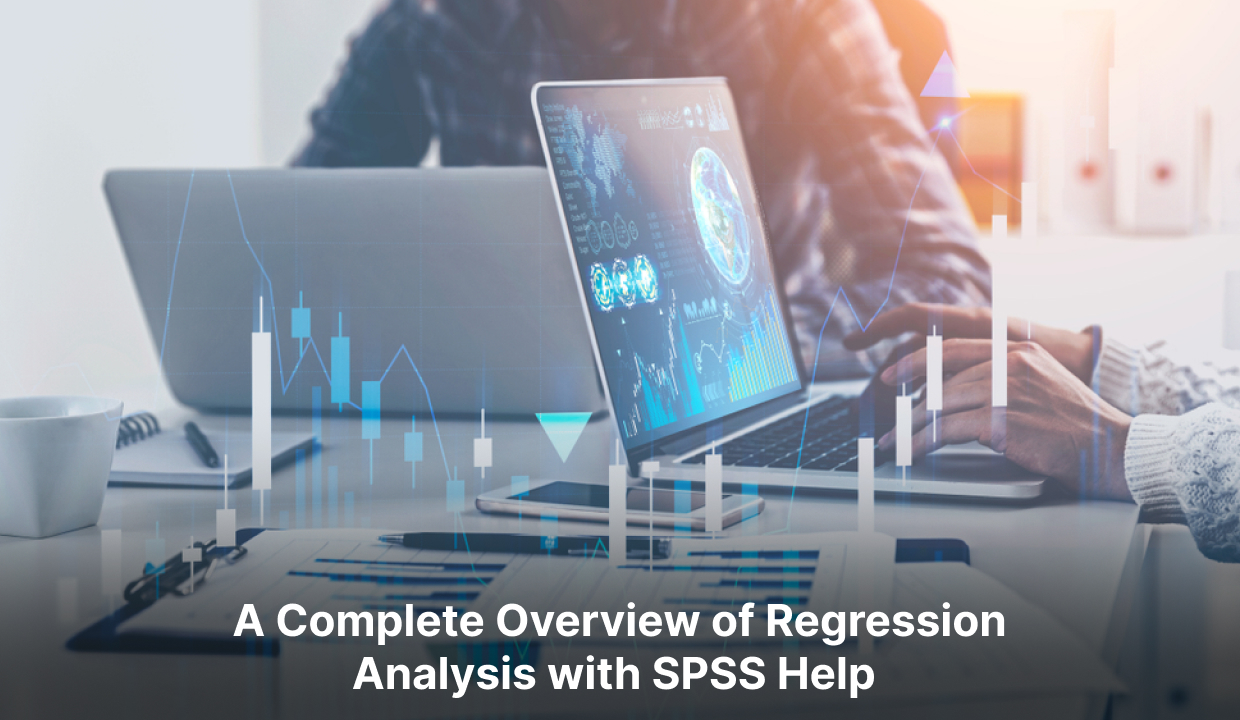
Contents
- Introduction
SPSS and SPSS Help, are widely utilised software and support in social sciences and business research, and are renowned for their expertise in data analysis. Among its valuable tools, regression analysis is a key analytical technique that aids researchers in comprehending variable relationships and making predictions.
Regression analysis, a powerful statistical method, holds immense significance across numerous disciplines such as economics, social sciences, finance, and data science. Whether you are a student, researcher, or data professional, possessing a firm grasp of regression analysis is crucial for making informed decisions and drawing accurate conclusions from data. This blog post delves deeper into the intricacies of regression analysis, specifically focusing on how companies effectively harness their potential.
What is Regression Analysis?
Regression analysis is a statistical method to explore the correlation between a dependent variable and one or multiple independent variables. Its purpose is to gain insights into how variations in the independent variables influence the dependent variable. The dependent variable is the one we want to predict or explain, while the independent variables are the factors potentially influencing the dependent variable.
The primary goal of regression analysis is to create a mathematical model that can accurately predict or explain the dependent variable’s behaviour based on the independent variables’ values. This model is usually represented by an equation describing the variables’ relationship. Regression analysis provides valuable insights into the strength and direction of the relationships between variables, allowing researchers to make predictions and understand the impact of different factors. It is widely used in various fields, including economics, finance, social sciences, and business, to analyse and interpret data, identify trends, forecast outcomes, and make informed decisions.
Types of Regression Analysis
-
-
-
Linear Regression
-
-
Linear Regression is a statistical technique that aims to establish a linear association between a dependent variable and one or multiple independent variables.
-
-
-
Multiple Regression
-
-
It involves various independent variables to predict the dependent variable.
-
-
-
Polynomial Regression
-
-
It models the relationship between the dependent and independent variables using polynomial functions.
-
-
-
Logistic Regression
-
-
It is used when the dependent variable is categorical or binary, and it predicts the probability of an event occurring.
-
-
-
Ridge Regression
-
-
It is a variant of linear regression that adds a penalty term to reduce the impact of multicollinearity among independent variables.
-
-
-
Lasso Regression
-
-
Similar to ridge regression, it adds a penalty term and performs feature selection by shrinking some coefficients to zero.
-
-
-
Elastic Net Regression
-
-
It combines the features of ridge and lasso regression by adding both L1 and L2 penalties to the loss function.
-
-
-
Time Series Regression
-
-
It analyses the relationship between variables over time to make predictions or forecast future values.
-
-
-
Nonlinear Regression
-
-
It models the relationship between variables using nonlinear functions, allowing for more complex relationships.
-
-
-
Stepwise Regression
-
-
A variable selection method that systematically selects and removes independent variables based on their statistical significance.