Takeaways for qualitative research and data analysis
While these thematic analysis examples span various fields within the social sciences, they share common methodological threads that highlight best practices in conducting effective thematic analysis. These commonalities can serve as valuable pointers for researchers aiming to employ thematic analysis in their work, regardless of their specific domain of study.
Systematic data engagement: Across all examples, a systematic approach to qualitative analysis is fundamental. Researchers personally engage in data collection and immerse themselves in the resulting data through repeated readings, enabling a deep familiarity to identify themes relevant to the data and research question. This immersion facilitates the initial coding process, where researchers employ data coding to capture data features relevant to the research questions. Researchers should approach their data systematically, ensuring thorough and consistent engagement to capture the depth and breadth of themes.
Iterative theme development: The examples illustrate that identifying themes is an iterative process. Preliminary themes are generated from initial codes that cluster similar data segments. These themes are then reviewed and refined, ensuring they accurately represent the data set. Researchers should be prepared to revisit their data and themes multiple times, refining their themes to ensure they are coherent, distinct, and meaningful. This iterative process is central to the rigor of thematic analysis.
Richness and complexity of themes: Thematic analysis, as demonstrated in these examples, excels in capturing the richness and complexity of qualitative data. Themes are not just surface-level categories; they encapsulate intricate patterns that provide deep insights into the data. Researchers should strive for generating themes that capture the complexity of their data, offering rich, detailed, and nuanced interpretations.
Contextual understanding: Each example underscores the importance of understanding the context from which the data are derived. Context shapes the data and the themes that emerge from them, influencing how researchers interpret and understand the identified patterns. Effective thematic analysis requires researchers to be acutely aware of their data’s contextual backdrop, integrating this understanding into their analysis and interpretation.
Transparency and rigor: These examples demonstrate the need for rigor throughout the thematic analysis process. This includes maintaining detailed records of the process of coding data, providing clear definitions for each theme, and offering comprehensive explanations of how themes were derived from the data. Researchers should document their analytic decisions meticulously, ensuring their analysis is transparent and credible.
Triangulation and validation: These hypothetical studies exemplify the value of triangulating thematic analysis findings with other data sources, theories, or methods to enhance credibility. Researchers should consider using additional data sources or analytical methods to validate and enrich their thematic analysis findings, ensuring robust and trustworthy conclusions.
Reflective practice: Finally, these examples highlight the importance of a reflexive thematic analysis. Researchers must continually reflect on their own contexts, assumptions, and perspectives, considering how these might influence their analysis. By engaging in reflective practice, researchers can mitigate potential biases, enhance analytic rigor, and ensure their findings are a credible representation of the data.
These common threads across different thematic analysis examples provide a foundation for conducting robust and insightful thematic analysis. By adhering to these best practices, researchers can leverage thematic analysis to yield meaningful, impactful insights from their qualitative data.
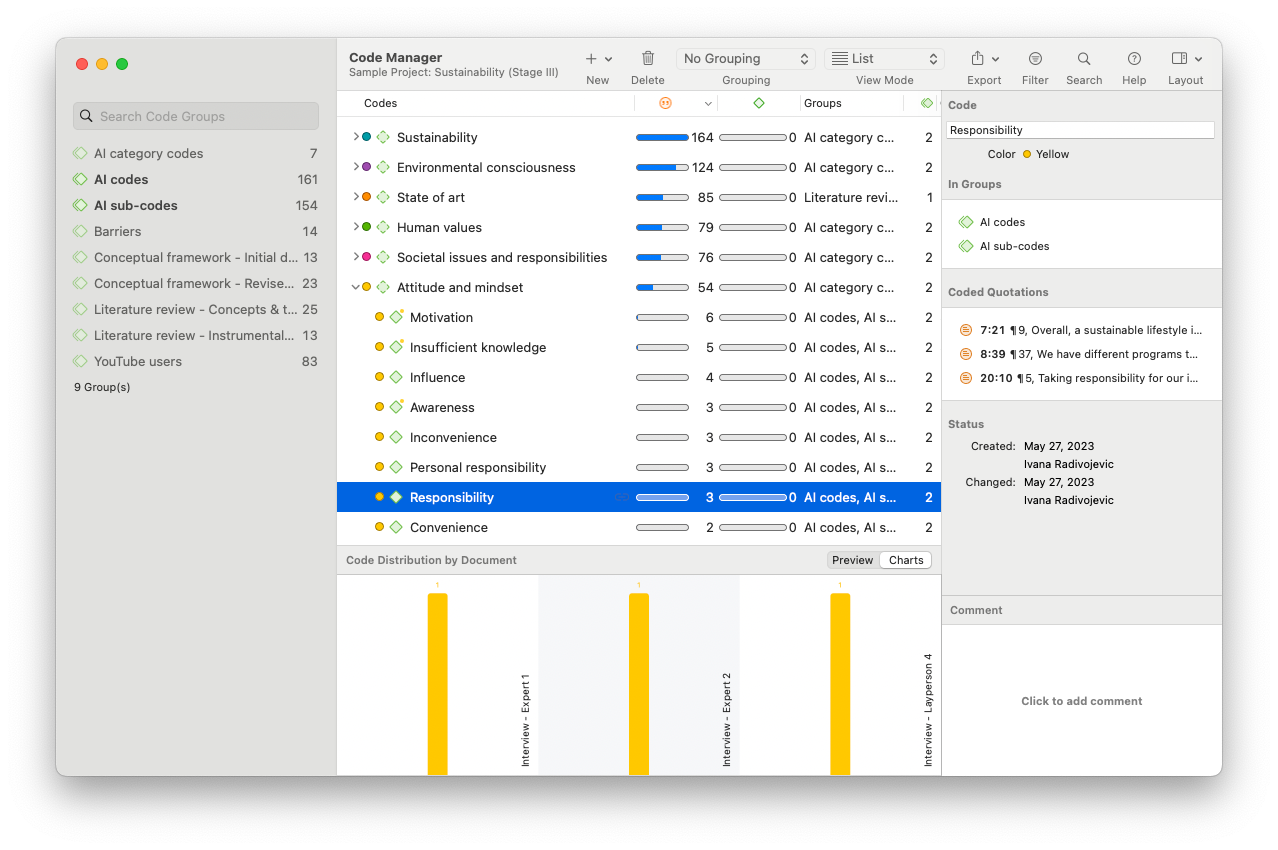