What can data analysis software do?
What can data analysis software do?
Data analysis software provides a range of functionalities that streamline and enhance the data analysis process. These tools help users manage data more effectively, retrieve relevant information quickly, and present findings in a clear and visually appealing manner.
This section will explore three key capabilities of data analysis software: data organization, data retrieval, and data visualization.
Data organization
One of the fundamental tasks in data analysis is organizing the data in a way that makes it accessible and easy to work with. Data analysis software helps with this task by providing tools that allow users to categorize, sort, and structure data according to various parameters. Whether dealing with qualitative or quantitative data, these tools enable users to manage large datasets efficiently.
For qualitative data, such as interview transcripts or survey responses, the software can help organize the data into themes, categories, or codes. This organization is critical for researchers who need to sift through large amounts of text to identify patterns or recurring themes. By grouping related data together, the software simplifies the analysis process and makes it easier to draw meaningful conclusions.
Quantitative data, such as numerical datasets, also benefits from the organizational features of data analysis software. The software can sort data by variables, filter out irrelevant information, and aggregate data points to provide a clearer picture of the overall trends. This level of organization is particularly useful when dealing with complex datasets that require careful management to ensure accurate analysis.
Data retrieval
Once data is organized, the next challenge is retrieving relevant information quickly and efficiently. Data analysis software offers robust search and retrieval functions that allow users to locate specific data points or subsets of data with ease. This capability is especially important in large datasets, where manually searching for information would be time-consuming and prone to error.
For qualitative data, retrieval features may include keyword searches, code searches, and the ability to retrieve data based on specific criteria, such as date ranges or respondent characteristics. These tools enable researchers to focus on the most relevant parts of the data, speeding up the analysis process and ensuring that no important information is overlooked.
Quantitative data retrieval is also enhanced by software tools that allow for the quick extraction of relevant data subsets. Users can apply filters, create custom queries, and generate reports that focus on specific variables or data points. This ability to retrieve data efficiently is key to conducting thorough and accurate analyses, particularly when working with large and complex datasets.
Data visualization
Data visualization is another key feature of data analysis software that plays an important role in interpreting and presenting data. Visualization tools transform raw data into visual formats, such as charts, graphs, and maps, making it easier to understand patterns, trends, and relationships within the data. This visual representation is not only helpful for the analyst but also for communicating findings to others, whether in a report, presentation, or publication.
Qualitative data can be visualized through tools that create word clouds, thematic maps, or flow charts, helping to illustrate the connections and themes that emerge from the data. These visual aids can make complex qualitative findings more accessible and easier to grasp.
For quantitative data, many visualization options are also available. Users can create bar charts, line graphs, scatter plots, pie charts, and other types of graphs to represent numerical data. These visuals can highlight key trends, compare variables, and show relationships between different data points. The ability to customize these visualizations—adjusting colors, labels, scales, and other elements—further enhances the clarity and impact of the presentation.
Moreover, some advanced data analysis software includes data visualization capabilities that allow users to explore their qualitative and quantitative data dynamically. For example, users might click on a part of a graph to drill down into more detailed data or adjust filters to see how different subsets of the data affect the outcomes. This interactivity can provide deeper insights and make data exploration more engaging and informative.
Types of data analysis software
Data analysis software comes in various forms, each tailored to specific types of data and analytical needs. Broadly, these tools can be categorized into two main types: qualitative data analysis software and quantitative data analysis software. Understanding the differences between these types is important for selecting the right tool for your research or business needs.
Qualitative data analysis software
Qualitative data analysis software is designed to handle non-numerical data, such as text, audio, video, and images. This type of software is particularly useful in fields like social sciences, education, and market research, where understanding the meaning, context, and themes within the data is more important than numerical analysis.
One of the key functions of qualitative data analysis software is coding, which involves labeling sections of data with tags or codes that represent specific themes or concepts. This process helps in organizing the data into categories, making it easier to identify patterns and draw conclusions. The software allows users to create and manage these codes efficiently, even when dealing with large volumes of data.
Another important feature of qualitative data analysis software is its ability to manage complex data types. For example, the software can handle transcripts of interviews, focus group discussions, or open-ended survey responses.
It also supports multimedia data, allowing researchers to analyze audio recordings, videos, and images within the same project. This flexibility makes it possible to perform in-depth analyses across various types of qualitative data.
Qualitative data analysis software also often includes tools for visualizing data. These tools can create visual representations of themes, code frequencies, or relationships between different codes, making it easier to interpret and present the findings. The ability to visualize qualitative data can be particularly helpful in communicating complex ideas to stakeholders or in academic publications.
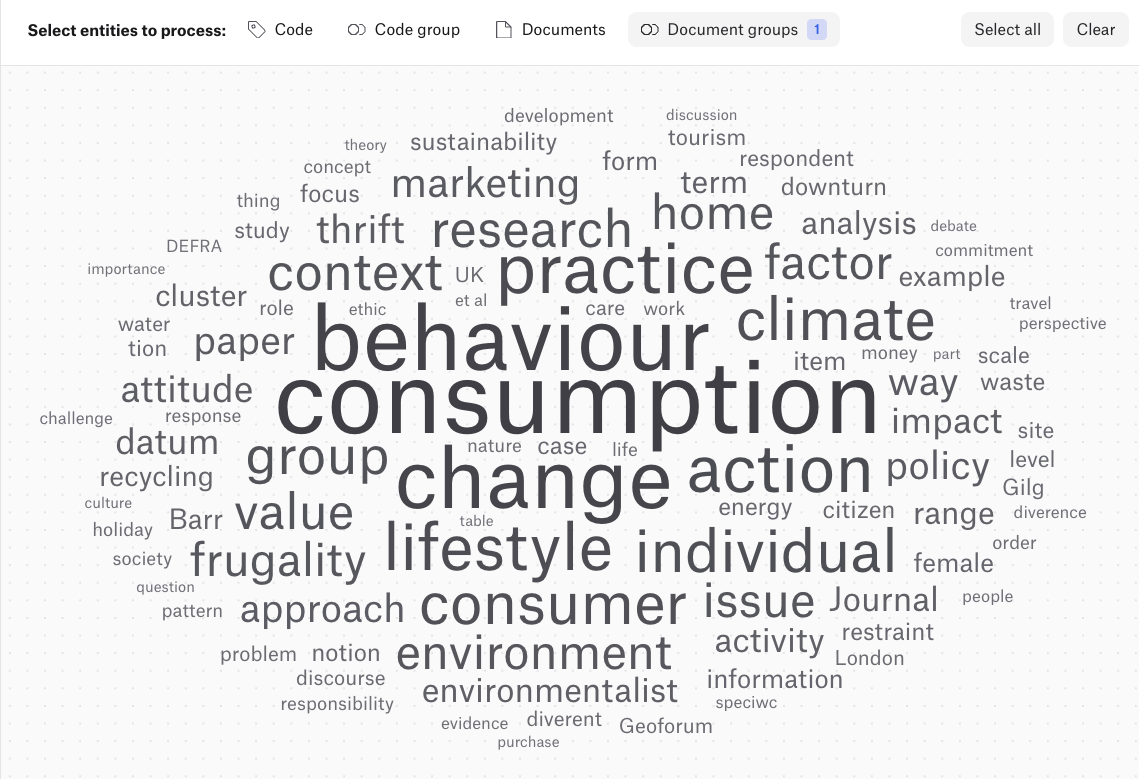
Quantitative data analysis software
Quantitative data analysis software is designed for numerical data. This type of software is commonly used in fields like finance, economics, engineering, and the natural sciences, where the focus is on numerical analysis, hypothesis testing, and statistical modeling.
One of the primary functions of quantitative data analysis software is statistical analysis. Statistical analysis software can perform a wide range of statistical tests, from basic descriptive statistics to more complex inferential analyses.
This includes tests like t-tests, ANOVAs, regression analyses, and chi-square tests, among others. The software automates these calculations, reducing the risk of human error and ensuring that the analyses are conducted accurately and efficiently.
Another key feature of statistical software is data visualization. Data manipulation for the purpose of visualization often includes sophisticated options, such as histograms, scatter plots, bar charts, and line graphs. These visualizations help users understand the distribution of data, identify outliers, and observe trends over time.
Quantitative data analysis software also typically includes features for data management, such as data cleaning and preparation. The software can identify and handle missing data, outliers, and other anomalies, ensuring that the dataset is ready for analysis. This is particularly important in quantitative research, where the accuracy of the data directly impacts the validity of the results.