What is the impact of sampling bias?
What is the impact of sampling bias?
Sampling bias can have profound and varied impacts on research, affecting everything from the validity of findings to the decisions made based on those findings. This section explores the major impacts of sampling bias, segmented into the validity of research, decision-making, and ethical considerations.
Validity of research
The most direct impact of sampling bias is on the validity of research findings. When a sample is not representative of the population, the results cannot be reliably generalized to a broader context. This lack of representativeness can lead to incorrect conclusions, misleading insights, and flawed theories.
For example, if a health study on a new medication excludes certain demographic groups, it might falsely conclude that the medication is universally effective, overlooking potential side effects or varying efficacies across different populations.
Decision-making
Decisions based on biased research can lead to ineffective or harmful policies, practices, and interventions. In healthcare, for instance, policy decisions about resource allocation or treatment guidelines that rely on biased studies might not address the needs of all population segments, potentially exacerbating health disparities.
In education, programs designed that are informed by research that overlooks underprivileged communities may fail to bridge the educational gap, further entrenching inequalities. The impact on decision-making underscores the importance of accurate, representative research for informing policies and practices that are equitable and effective.
Ethical considerations
Sampling bias also raises ethical concerns, particularly regarding fairness and equity. Research that consistently excludes or misrepresents certain groups contributes to their marginalization, reinforcing systemic biases and inequalities.
This not only affects the groups’ visibility in research but also their access to benefits derived from scientific advancements. Ethically, researchers have a responsibility to ensure their work inclusively reflects the diversity of society, promoting equity in knowledge creation and its applications.
Types of sampling bias
Sampling bias can manifest in various forms, each affecting the research outcome in different ways. Understanding these types is instrumental for identifying and mitigating potential biases in studies. This section outlines four common types of sampling bias and discusses stratified random sampling to help address some forms of sampling bias.
Survivorship bias
Survivorship bias occurs when a study focuses only on the subjects that “survived” or made it past a certain selection process, ignoring those that did not. This can lead to overly optimistic or skewed results.
For example, in analyzing the success of startups, focusing only on those companies that have thrived without considering the many that have failed could lead to an overestimation of the factors contributing to success. Recognizing survivorship bias helps researchers consider the full scope of data, including failures, to draw more accurate conclusions.
Observer bias
Observer bias arises when the researchers’ expectations or knowledge influence their observation and recording of data. This type of bias is particularly relevant in observational research or experiments requiring subjective interpretation.
For instance, if a researcher expects a certain outcome from a study, they might unconsciously interpret ambiguous responses to fit their hypothesis, skewing the results. In such cases, blinding methods or third-party data analysis can help mitigate observer bias.
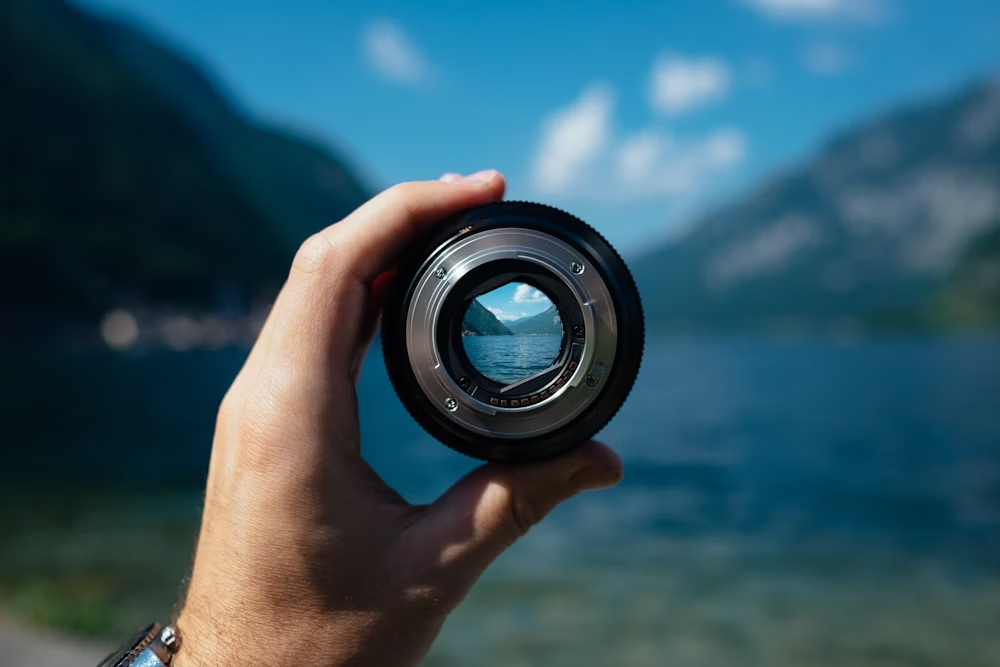